Bayes
Problem Set 3
贝叶斯作业代写 Problem 1: The mean problem with a prior on σ Fill in section 1.2 of Bayes Lecture Note 2 with a prior on σ in addition to the conjugate prior on μ.
- Submit the problem via GradeScope – indications will be emailed.
- To get a check, you need to answer allthe questions.
- All discussion and theoretical questions must be hand written to count.
- All R code must be shown at the end of the Problem Set
Problem 1: The mean problem with a prior on σ 贝叶斯作业代写
Fill in section 1.2 of Bayes Lecture Note 2 with a prior on σ in addition to the conjugate prior on μ. For σ use an inverted gamma prior with hyper-parameters (ν0, s0).
Write the joint posterior density of p(μ , σ | D).
Then show how to find the conditional posterior p( μ | σ , D), the posteriors p(σ | D), p(mu | D).
Write all the needed posterior parameters as function of sufficient statistics and prior hyper-parameters.
The answer to this problem must be hand written
Problem 2: Estimation and prediction by simulation 贝叶斯作业代写
You still remember this mid August 2015, you had just started your job as a quant. after graduating from the . On that week the VIX jumped from 12.8 last week to 28.03. The S&P 500 was down 6%. It was panic and all hands on deck. Everybody wanted to know what the VIX would do the following weeks.
Well you survived and here you are. Thinking back, you wonder if an autoregression would have helped forecast future volatility. You happen to have weekly data starting in 2011 in file vix-sp-week.csv. You use the data up to Aug. 17, 2015 included to estimate the model. Then you make predictions for the next three weeks.
You very well know that the proper formulation is in logarithms rather than levels. So specifically you consider the model
LVt = ϕ0 + ϕ1 LVt-1 + ϵt , ϵ ~ N( 0, σ ),
where LVt is the logarithm of VIX. You use a Bayesian regression which will allow to simulate by direct Monte Carlo methods the posterior densities of the parameters as well as the three predictive densities at T+1, T+2, T+3. You use lsfit to get the needed sufficient statistics.
You very well know that the better formulation for an AR(1) is in Log. Simulation will make it very easy to draw the predictive distribution of VIX (V) directly by transforming draws of Log(VIX), (LV) from a Log regression. So you estimate
LVt = ϕ0 + ϕ1 LVt-1 + ϵt , ϵ ~ N( 0, σ ),
with a Bayesian. Construct the X and the Y variable, where X0 is LV on 3/26/2019.

b)
So the likelihood uses data only from 2012. In Table 1, write the posterior means and posterior standard deviations of ϕ0, ϕ1, and the posterior parameters ν and s, and the mode and standard deviation of σ, computed analytically. Use AZ appendix as needed. Report two digits as in .xx
c)
Drats! Your boss reminds you that σ has a skewed distribution, so its standard deviation is not useful. She asks you to report the 25% and 75% quantiles of σ . You need to simulate σ to get these quantiles. Anyway you would have to simulate to generate predictive draws, so it’s not a problem. From here on, everything is based on 10,000 drawsof each relevant random variable.
As you are simulating, you can enforce the belief that ϕ1 ∈ [0,1[. You strongly believe that the VIX is stationary and positively autocorrelated. You will enforce the belief by rejecting negative or larger than 1 draws of p(ϕ1 |σ , D)
- Simulate σ|D.
- Simulate ϕs: How many draws did you have to simulate to keep 10000 draws?
d) 贝叶斯作业代写
Now build your predictive density simulation and prepare your Table 2 of results. First, report on the posterior and predictive densities of σ, ϕ1, E(V), V1, V2 , V3. You will simulate E(V) the mean of the VIX implied by (σ, ϕ0,ϕ1). The last three are the 1-week, 2-week, 3-week ahead VIX.
- Write the formula used for (E(V) | σ, ϕ ), the mean of VIX implied by the AR(1) parameters
- You don’t care about log(VIX), you care about VIX. But for each draw of log(VIX), you have a draw of VIX by simple transformation.
The rows of the table will be: Mean, Median, 25% and 75% quantiles, simply computed as the sample statistics of your 10,000 draws
- On Figure 1, show a times series plot, show VIX from Jan 2014 to Aug. 17, 2015, and then the five predicted VIX; predictive mean and 25%, 75% quantiles of VIX. Add the true values of the VIX for these 3 weeks.
- On Figure 2. Plot the three predictive densities of the 1-, 2-, 3 week ahead VIX.
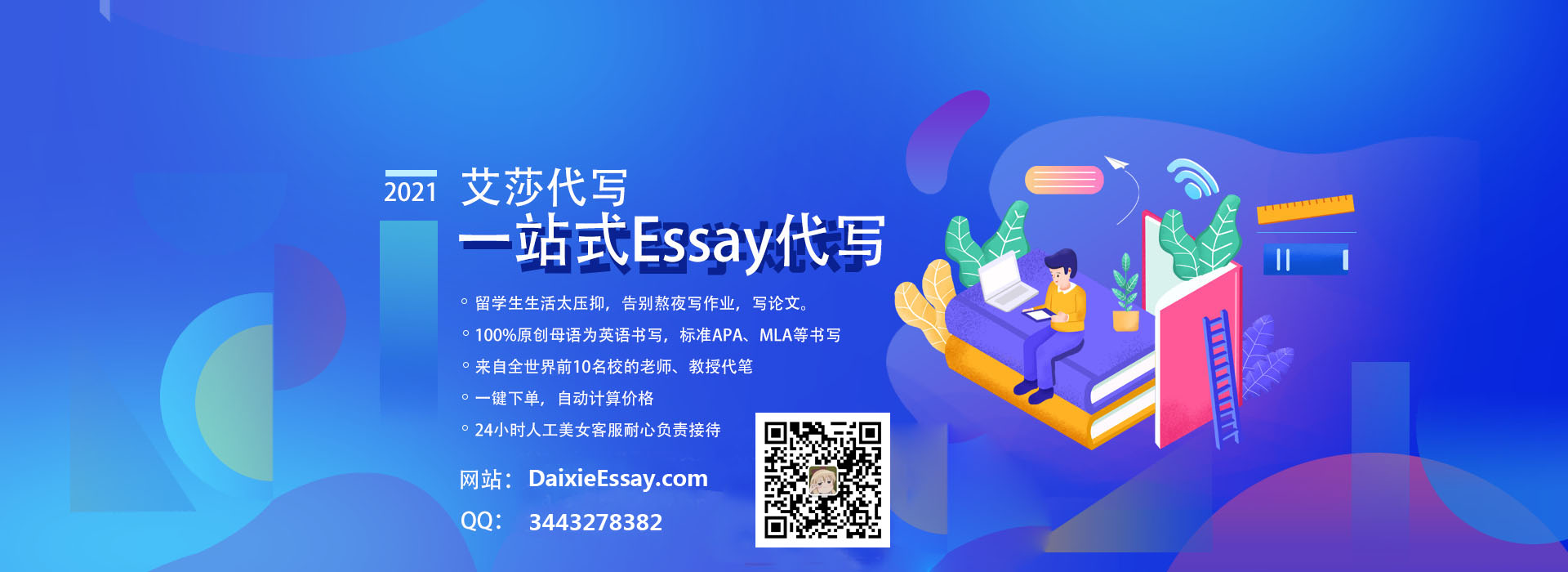
更多代写:r语言作业代写 宏观经济final quiz代考 英国法律Assignment代写 东亚和南亚研究essay代写 高中作业report代写 休闲学代写