Problem 1. [Estimating a Hilbert’s density] (12 pts, 4 ⇥ 3)
因编辑器不支持公式 所以乱码 pdf预览查看
Let {Xi}n be an i.i.d. sample from an unknown distribution P with (Lebesgue) density f supported on
some compact X ⇢ Rd with Lebesgue measure 1. For simplicity, we assume furthermore that f is bounded,
i.e., supx f(x) F for some known F. .
R, X g2 dx < 1}. Suppose we know an orthonormal basis {fj}1j=1 of F (under the inner-product hg, hi =
X g(x) · h(x) dx); then the idea is to estimate the expansion coefficients ↵j of f, where f = P1j=1 ↵jfj,
In particular, one idea is to minimize (an approximation to)
2 D E
fbN — f = kfk + kfbN k2 — 2 fbN ,f .
In particular, this is equivalent to minimizing kfbN k2 — 2hfbN ,fi, where kfbN k2 = PN ↵2, and where
hfbN ,fi R fbN (x)f(x) dx = EfbN (X) can be estimated from a sample as 1 Pn fbN (Xi). In other
N n
EkfN — fkp ———! 0, provided N = o(n),N ! 1.X ↵b2 — 2 X fb (X ). (1)
(1). Show that equation (1) is minimized by setting ↵bj = 1 Pn fj(Xi), for j = 1,. .., N.
- Show that fNso defined is consistent, i.e., for any 1 p 2, we have
- Supposethe tail coefficients of f satisfy 1j=N+1 2
.
Ø(N), for some known Ø(N)
2
—N——!—1! 0.
Derive an asymptotic L2 confidence ball of the form B6 = {g 2 F : kfbN — gk r6}, where r6 depends
on n, N, Ø(N), confidence level 1 — 6 (for any fixed 0 < 6 < 1), and z-critical values of N (0, 1).
Problem 2. [When is classification well-defined?] (8 pts, 2 ⇥ 4)
Let X be a random variable (⌦, ⌃) 7! (R, B(R)) with range R. In classification, a label y 2 {0, 1} is associated to every value x of X in R. Usually, we posit the existence of a labeling function h(x), h : R ! {0, 1}. If such h is not measurable (R, B(R)) 7! ({0, 1}, 2{0,1}), classification is not well-defined as a statistical problem, e.g., we cannot talk about estimating h under given Risk measures (e.g. P(h 6= h)).
- Usingthe fact that B(R) 6= 2R, argue that, without additional assumptions, a labeling function h might indeed not be measurable (R, B(R)) 7! ({0, 1}, 2{0,1}).
- SupposeY : (⌦, ⌃) 7! ({0, 1}, 2{0,1}) is jointly distributed with X (on (R2, B(R2))). Argue that, there always exists a labeling function h of the form {E[Y |x] ≤ 1/2} (so-called Bayes classifier), where h is measurable (R, (R)) ( 0, 1 , 2{0,1}).
Hint: The rights answers should be a few lines following from basic definitions and results.
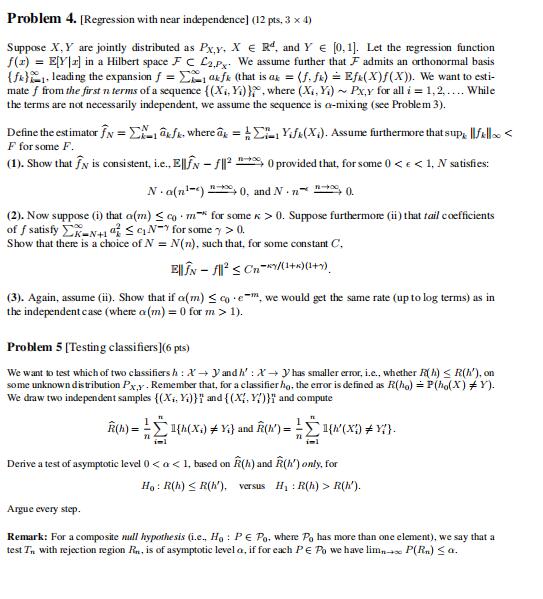

