The Bartlett School of Environment, Energy and Resources
Energy Adoption Analysis代写 By submitting this document, you are agreeing to the Statement of Authorship:I/We certify that the attached
MSc ESDA Coursework Title Page
UCL Candidate Code: ZSPZ6
Module Code: BENV0092
Module Title: Energy Analytics in the Built Environment
Coursework Title: An Analysis of Household Cooking Energy Adoption
Module Leader: Despina Manouseli
Date: 09/01/2019
Word Count: 2642
By submitting this document, you are agreeing to the Statement of Authorship:
I/We certify that the attached coursework exercise has been completed by me/us and that each and every quotation,diagram or other piece of exposition which is copied from or based upon the work of other has its source clearlyacknowledged in the text at the place where it appears.
I/We certify that all field work and/or laboratory work has been carried out by me/us with no more assistance fromthe members of the department than has been specified.
An Analysis of Household Cooking Energy Adoption
1. Introduction Energy Adoption Analysis代写
When it comes to energy services of a residential building, we mostly look into heat and electricity, while neglecting some more fundamental services like cooking. Clean, reliable and affordable cooking fuel supply is the very basic need of people’s life and human welfare. Researchers from developed countries might take it for granted, but in most developing countries, it remains an issue to be addressed. In the case of China, cooking activity consumes more than 40% of total domestic energy consumption in rural regions (China Academy of Development and Strategy, 2015).
Although there is a wide range of household’s cooking fuel choice,
from solid fuels (biomass and coal) to modern fuels (LPG, pipeline gas, electricity and biogas) which are much cleaner, many families suffer from healthy and environmental challenges raised by using solid fuels indoors. The household air pollution (HAP) from incomplete combustion of cooking fuels is responsible for 1.5 million underage death and one third of upper respiratory infections (World Health Organisation, 2016b) and most victims are women and kids. Also, cooking fuel choice makes another indicator of “fuel poverty” in many developing countries (Quinn et al., 2018).Energy Adoption Analysis代写
This essay tries to analyse household’s shift to clean cooking fuels from the scope of people. Section 2 listed some key theories and related literature to fuel choice and modelling. Section 3 introduces the raw data set and logistic regression which is adopted for the analysis of Section 4. The forth part focused on interpretation of the key economic and social variables and regression results. Conclusions and limitations are discussed in the fifth part.
2. Literature Review
The theoretical frame of studies on fuel choices originates from two models: energyladder and fuel stacking. The energy ladder model attributes differences in energy consumption choices among households to variations in family wealth. It argues that solid fuels, like straws and charcoal, are inferior energy for low-income groups, and these groups will switch to a more “advanced” fuels (like gas) step by step when their family wealth increases.
Such process can be imagined as climbing up a dummy “ladder”. Many early-age studies based on this model value family wealth as a key role in modelling household’s cooking fuel shifts like those of Hosier and Dowd (1987). The deficiencies of energy ladder theory come from exclusion of demographic and social determinants like household characteristics and infrastructure situations. Besides, more discussion are needed on whether it is reasonable to divide into inferior and superior and associate them with specific groups (Akpalu, Dasmani and Aglobitse, 2011).Energy Adoption Analysis代写
Similar to energy ladder, fuel stacking also emphasizes the process of fuel shift with family wealth, but argues that households do not simply “jump” from one fuel to another as their wealth accumulates, but gradually transitions to another fuel. This continuous change explains the fact that only a few household depends on one specific fuel, whereas many households in developing countries keep mixed fuels for a long period. For example, many households in Ethiopia have Kerosene and biomass in stock at the same time as cooking fuels (Alem et al., 2016).
Households with mixed fuels have more resistance against fuel shortage and fluctuating fuel price.
Another feature that distinguishes fuel stacking from energy ladder model is that it does not define the direction of cooking fuel shifts as family wealth accumulates. In fact, households sometimes change from “high-income” fuel to “inferior” fuel, and this preference for a specific fuel type have cultural and social backgrounds. For example, many households in urban India would still keep the wood oven when refurbishing their kitchen. According to Rosenthal et al., 2018, the increased wealth is more likely to allow households keep more fuel types rather than totally switch to modern and cleaner cooking fuels (2018).Energy Adoption Analysis代写
Based on the two models mentioned above, further studies included other household characteristics, of which education level of family members has drawn particular attention. Higher education level helps to better understand the technology and environment background of specific fuel and make more “rational” decision on this basis. Also, better educated household members are more aware of the environmental and healthy risk of traditional solid fuels and more open to switch to cleaner fuels. With higher education level, household members spend more opportunity cost collecting and consuming solid fuels, which pushes them to clean fuels which are more convenient and much easier to use.
3. Methodology Energy Adoption Analysis代写
3.1 Data
The raw data set came from four waves of China Family Panel Studies (CFPS) launched by the Institute of Social Science Survey of Peking University, China from 2010 to 2016. The first wave of household survey in 2010 covered 15000 families and 30000 individuals across China, and many new households joined in the following waves which were conducted every other year. The questionnaire classified household’s cooking fuel choices into seven categories: Firewood/Straw, Coal, Gas/Liquid, Natural Gas, Solar energy, Electricity and Other and participants were only asked to choose one cooking fuel that is primary in their daily life.
Fig. 1 shows the overall trend of household cooking fuel adoption from 2010 to 2016.
With declining proportion of solid fuel and growing share of gas and electricity, the fuel structure of Chinese households have been improving since 2006, due to economic growth and urbanisation. The CFPS data set also provided comprehensive evaluation of family characteristics with more than 2000 variables on levels of individual, household and community and makes an excellent raw data source to look into
people’s behavior in energy services and underlying social norms. This essay only focus on people’s role in energy service on a household level, so a subset with about 600 variables were selected from the data set.Energy Adoption Analysis代写
Fig. 1 Household cooking fuel adoption from 2010 to 2016
One issue with CFPS data set is that it does not include the fluctuation of fuel price during the survey and other fuel properties. Therefore, fuel poverty associated to cooking can not be fully evaluated. The survey questions were also designed to only look at the primary cooking fuel in households, thus automatically eliminating the impact of fuel stacking and simplifying the process of cooking fuel switch.
3.2 Model
The model bases on the “utility maximization theory” that a household will abandon the cooking fuel i in use for the moment only if the utility of another cooking fuel j exceeds the that of the current one (Yang and Sun, 2012). In other words, if a household is to decide which kind of fuel to adopt, it always chooses the fuel source which provides the largest utility. The fuel’s utility is expressed in the form of linear combination of variables from the data set plus an unobserved error term. This is written as:
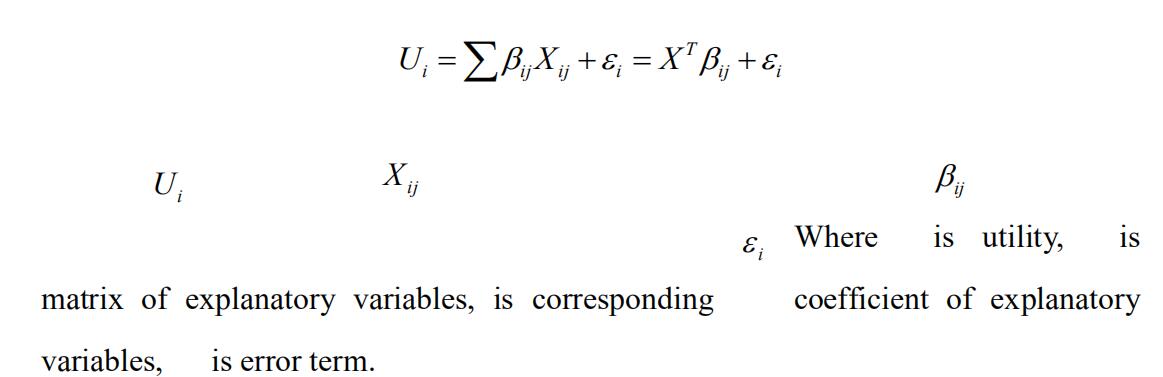
When the utility is higher than a threshold, the probability of households’ choosing clean energy are higher than the probability of households’ staying with solid fuels and the household will thus switch to clean energy. It is also assumed that the switching process is discrete, with no transition levels where two or more fuels coexist. With reference to classification regression, the log odds of choosing clean fuel over solid fuel can be expressed as:
chooses clean cooking fuels, and otherwise,
It is worth reminding that solid fuels include biomass and coal, whereas clean fuels or modern fuels mean LPG, natural gas, electricity biogas and solar.
4. Analysis and results Energy Adoption Analysis代写
4.1 Variable Descriptions
(a)Urbanvariable
Urban variable is a dummy variable to indicate if one household is urban or rural, with two levels (0 for rural area and 1 for urban area). This variable describes the variation between rural and urban regions in infrastructure construction, transport energy accessibility and social norms. But whether a household belongs to rural or urban is decided not by household registration records, but by if its family members engage in agricultural production. The reason for choosing such division is to acknowledge that farming households spend a lower opportunity cost in accessing and collecting biomass.Energy Adoption Analysis代写
From Fig. 1 we can see that rural and urban households have very distinct cooking fuel structures. In year 2010, clean energy had dominance in urban households’ cooking consumption while in rural regions, were adopted by only 25% households. From 2010 to 2016, biomass remained primary cooking fuel among rural households, but its proportion has dropped by 17%. Although the rural-urban gap still remained, Fig. 1(b) shows a much improved cooking fuel structure in Chinese households, especially in rural regions.
(a)
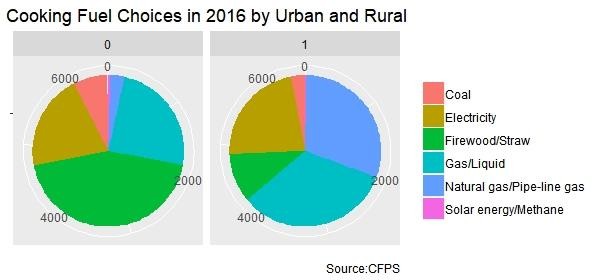
(b)
Fig. 1 Household cooking fuel adoption by urban and rural division in (a) 2010 and (b) 2016
The dummy variable “0” refers to rural areas and “1” refers to urban areas.
(b)Familymembers Energy Adoption Analysis代写
Family member decides the base demand for cooking fuels and helps to identify the different behavior patterns among single and married, childless or with child
households. There were also studies looking for fuel stacking behaviours by household sizes, finding that large households adopts more mixed cooking fuels (Heltberg, 2004). Households with more than 8 members are removed. As has shown in Fig. 2 the most common family size is 3 to 5 in both rural and urban regions, but there are more “large families” living in urban.
Fig. 2 Family size in urban and rural regions in 2010
(c)Education
Education variable represents the highest education level among all family members. This variable has 8 values in the original data source, but is simplified to 3 values in the model in section 4.2, with 1 representing below junior high, 2 representing below bachelor and 3 representing the rest. Someone believes that members with higher education level are more aware of benefit in the environment and human body of clean fuels and are since more active in cooking fuel shift.Energy Adoption Analysis代写
But others are quitesceptical about the impact of education because there is also an observed gap between knowledge and actual behavior. Fig. 3, shows a declining trend of solid fuels and rising trend of modern fuel as the education level increases in both rural and urban households. The distinction between different education groups are more distinct in rural families with an observed 43% decrease in solid fuel adoption.
(a)
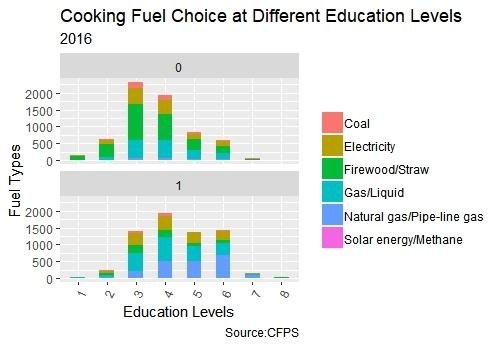
(b)
Fig. 3 Households’ cooking fuel adoption by education levels in (a) 2010 and (b) 2016
The figure in the x axis represents the highest education level of all household members, with 1 representing the lowest level (Illiteracy/ Semi-lliteracy) and 8 representing the high level (Doctor’s degree).
(d)Income
Both energy ladder and fuel stacking theory mentioned above highlight households’ wealth as a major constrain of their adoption of cooking fuels. The wealth determinant is significant in switching from solid fuel to clean fuel when it takes “initial capital” to install pipelines and stoves and “working capital” to purchase fuels and maintain the facilities (Goldemberg, 2000). There are some studies discussing which metric best evaluates household wealth (Hou, Liao and Huang, 2018).
Both household income and household asset can reflect family wealth from different scopes.
In view of the overall wealth level of a household fluctuates with economic development of the region and country, it would be better to consider the relative level of a specific household in the whole participants than use the absolute value of a family’s wealth. The wealth variable is created to show the position of a household in the quintile of the whole data set. That is to say, the wealth variable has 5 levels, ranging from 1 to 5,Energy Adoption Analysis代写
representing the poorest, median poor, median, median rich and richest quintile of the group respectively. From Fig. 4 we try to find a better segmentation metric of household wealth and use the variable for regression. All three metrics identify richest and median quintile groups quite well, with obvious distinction observed in cooking fuel structure. But asset and income plus asset metrics give a rather fuzzy segmentation of median and poorest groups, especially in the case of asset, the solid fuel proportion of median and poorest are both around 58%. The income metric is chosen to generate the household wealth variable, which will be used in logistic regression.
(a)
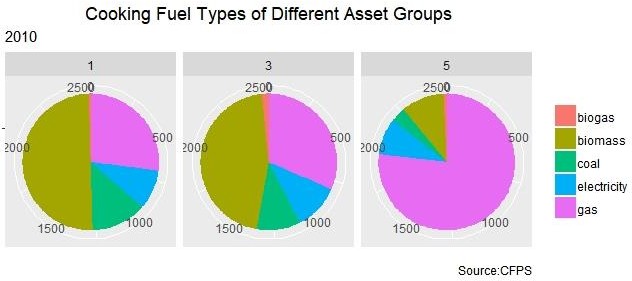
(b)
(c)
Fig. 4 Household cooking energy adoptions by (a) income (b) asset (c) wealth groups in 2010
4.2 Logistic RegressionEnergy Adoption Analysis代写
If we look at the AIC decrease shown in Table. 1, the model is improved by adding variables that evaluate household characters from different scopes. The interpretability of the model is strengthened after adding education level variable and urban-rural indicator. The coefficients in table. 2 quantify the log-odds of switchingto clean cooking fuel of a specific household.
The coefficients of quintile group variables are all positive,indicating that households with a higher level of income (compared to group 1) are more likely to adopt clean cooking fuels. Similarly, the positive coefficients of education variables show that household members who received higher level of education, are more likely to depend on modern energy sources.Energy Adoption Analysis代写
The family size variable is a bit complicated.
The log-odds of adopting clean fuel increase for 3-people household versus households with only 1 person. But there are two statistically insignificant levels which are level 2 and 6. From Fig. 2 we can see that it can not be attributed to small sample numbers, because households with 2 and 6 members takes a large proportion. Probable reasons could be sparsity of energy adoption within these two groups or family size is not a good metric for family structure.
Table.1 Variables and AIC decreases
Model | Variable(s) included Energy Adoption Analysis代写 | AIC decreases (compared to model 1) |
1 | Household income | / |
2 | Household income+familysize | 565 |
3 | Household income+education | 703 |
4 | Household income+familysize+education | 1307 |
5 | Household income+urban indicator | 5182 |
6 | Household income+ familysize+ education+ urban indicator | 5760 |
Table. 2 Regression results
Energy Adoption Analysis代写 | Estimate | Std.Error | z value | Pr(>|z|) |
(Intercept) | -2.273e+02 | 1.027e+01 | -22.128 | < 2e-16 *** |
quintile_income2 | 1.129e-01 | 4.818e-02 | 5.215 | < 2e-16 *** |
quintile_income3 | 5.881e-01 | 4.853e-02 | 11.633 | < 2e-16 *** |
quintile_income4 | 9.286e-01 | 4.906e-02 | 15.131 | < 2e-16 *** |
quintile_income5 | 2.098e+00 | 5.009e-02 | 17.130 | < 2e-16 *** |
familysize2 | 6.171e-02 | 2.848e-02 | 1.155 | 0.248041 |
familysize3 | 4.413e-01 | 5.348e-02 | 8.252 | < 2e-16 *** |
familysize4 | 1.932e-01 | 5.408e-02 | 3.572 | 0.000354 *** |
familysize5 | 1.496e-01 | 5.579e-02 | 2.681 | 0.007331 *** |
familysize6 | 3.294e-02 | 6.000e-02 | 0.549 | 0.582955 |
best_edu2 | 4.089e-01 | 3.030e-02 | 13.495 | < 2e-16 *** |
best_edu3 | 3.476e-01 | 2.967e-02 | 11.717 | < 2e-16 *** |
fk1l1 | -1.632e+00 | 2.536e-02 | -64.324 | < 2e-16 *** |
Significance codes: 0 ‘***’ 0.001 ‘**’ 0.01 ‘*’ 0.05 ‘.’ 0.1 ‘ ’ 1
5. Conclusion Energy Adoption Analysis代写
This essay gives some insight into household’s cooking energy consumption by identifying key economic and demographic variables. Household income, education level of occupants, family size and urban variables are significant predictors of household’s cooking fuel adoptions. Although cooking fuel structure has been improving in the past few years, large dependence on solid fuels remain a challenge to indoor air quality and household energy efficiency in developing countries. Knowledge, money and infrastructure are key constrains to shift to a sustainable
cooking in households.Energy Adoption Analysis代写
In the logistic regression, some issues with family size variable was discussion in Section 4.2, but it still deserves considering if there are other variables that can better describe family structure. In terms of spatial imbalance, while this essay only introduced urban-rural variable, there are more detailed district segmentation methods which are worth exploring. And the data set that is used in this essay did not include fuel’s price and energy density, which makes it hard to evaluate cooking fuel poverty.
References
Akpalu, W., Dasmani, I. and Aglobitse, P. (2011). Demand for cooking fuels in a developing country: To what extent do taste and preferences matter?. Energy Policy, 39(10), pp.6525-6531.
Alem, Y., Beyene, A., Köhlin, G. and Mekonnen, A. (2016). Modeling household cooking fuel choice: A panel multinomial logit approach. Energy Economics, 59, pp.129-137.
China Academy of Development and Strategy (2015). Chinese Household Energy Consumption Report 2015.Energy Adoption Analysis代写
Goldemberg, J. (2000). World energy assessment. New York: UNDP.
Heltberg, R. (2004). Fuel switching: evidence from eight developing countries. Energy Economics, 26(5), pp.869-887.Energy Adoption Analysis代写
Hosier, R. and Dowd, J. (1987). Household fuel choice in Zimbabwe: an empirical test of the energy ladder hypothesis. Resources and Energy, 9(4), pp.347-361.
Hou, B., Liao, H. and Huang, J. (2018). Household cooking fuel choice and economic poverty: Evidence from a nationwide survey in China. Energy and Buildings, 166, pp.319-329.
Quinn, A., Bruce, N., Puzzolo, E., Dickinson, K., Sturke, R., Jack, D., Mehta, S., Shankar, A., Sherr, K. and Rosenthal, J. (2018). An analysis of efforts to scale up clean household energy for cooking around the world. Energy for Sustainable Development, 46, pp.1-10.
Rosenthal, J., Quinn, A., Grieshop, A., Pillarisetti, A. and Glass, R. (2018). Clean cooking and the SDGs: Integrated analytical approaches to guide energy interventions for health and environment goals. Energy for Sustainable Development, 42, pp.152-159.
Yang, W. and Sun, B. (2012). Measurement and Decomposition of Household’s Vulnerability in Rural China. Economic Research Journal, 4, pp.40-51.
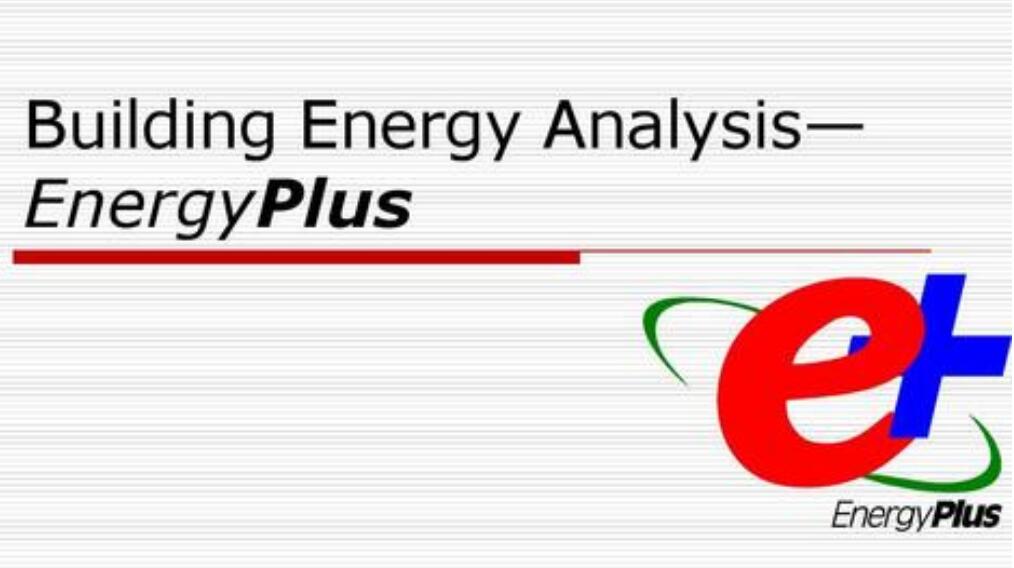
更多其他:C++代写 java代写 r代写 代码代写 金融代写 物理代写 考试助攻 C语言代写 finance代写 code代写 lab代写 计算机代写 code代写 data代写 Data Analysis代写