Qualifying Exam: CAS MA575, Linear Models
Linear Models代写 where β = (β1, . . . , βp)T Rp is the regression coefficient to be estimated. Instead of using ordinary least squares
Boston University, Fall 2016
1.Consider the linear regressionmodelLinear Models代写
Y = Xβ + e,
where β = (β1, . . . , βp)T Rp is the regression coefficient to be estimated. Instead of using ordinary least squares, an alternative is to consider the ridge estimator
β˜ = (XTX + λIp×p)−1XTY ,Linear Models代写
where λ 0 is a tuning parameter prespecified by the user and Ip×p is the identity matrix in Rp×p. Throughout this problem, assume that the design matrix X is deter- ministic and that the errors are independent normal random variables with mean zero and variance σ2 > 0.
- FindE(β˜). When is β˜an unbiased estimator of β?
- Givena data, explain the behavior of β˜ when λ , namely when an extremely large tuning parameter is
- Findthe covariance matrix of β˜.Linear Models代写
- Suppose σ2= 1 is known and one is interested in testing if β1 = 0. Is it possible to achieve this by using the ridge estimator β˜? Explain your strategy in detail (including the form of your test statistic and the distribution that you will use to obtain the cut-off value).
- Suppose σ2= 1 is known and one is interested in testing if β1 = β2 = = βp = 0. Is it possible to achieve this by using the ridge estimator β˜? Explain your strategy in detail (including the form of your test statistic and the distribution that you will use to obtain the cut-off value).
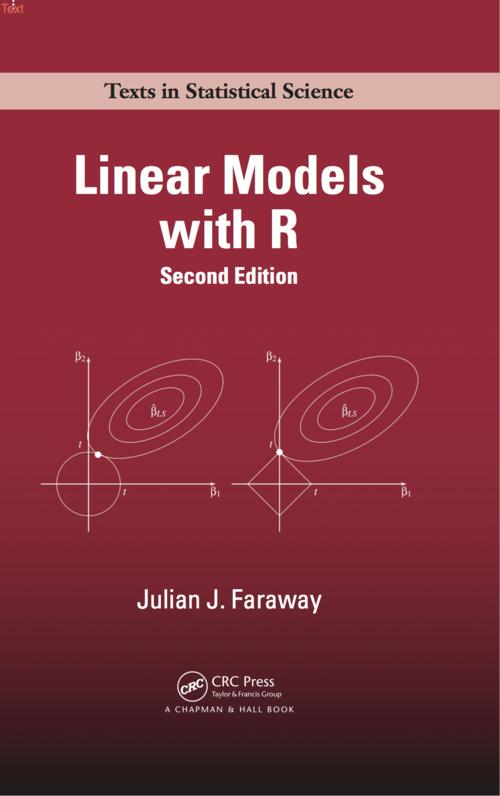
2.Consider the followingdataLinear Models代写
Degree | Response |
Undergraduate | 37.7, 32.5, 34.1 |
Master Linear Models代写 | 27.8, 22.7, 31.6, 36.5, 41.3 |
Doctoral | 38.2, 44.6, 35.4, 33.7, 40.2 |
The associated R output of regressing the response on the degree variable is given below.
Call:
lm(formula = Y ~ Degree)
Residuals:
Min 1Q Median 3Q Max
-9.280 -3.020 -0.380 2.933 9.320
Coefficients:Linear Models代写
Estimate Std. Error t value Pr(>|t|)
(Intercept) 38.420 2.441 15.737 2.2e-08 ***
DegreeMaster -6.440 3.453 -1.865 0.0917 .
DegreeUndergraduate -3.653 3.987 -0.916 0.3810Linear Models代写
—
Signif. codes: 0 *** 0.001 ** 0.01 * 0.05 . 0.1 1
Residual standard error: 5.459 on 10 degrees of freedom
Multiple R-squared: 0.2589, Adjusted R-squared: 0.1107
F-statistic: 1.747 on 2 and 10 DF, p-value: 0.2235Linear Models代写
Residual standard error: 5.459 on 10 degrees of freedom Multiple R-squared: 0.2589, Adjusted R-squared: 0.1107
F-statistic: 1.747 on 2 and 10 DF, p-value: 0.2235
Let
β1 = E(Response | Degree = Undergraduate)
β2 = E(Response | Degree = Master)
β3 = E(Response | Degree = Doctoral)
Throughout this problem, assume that the errors are independent normal random vari- ables with mean zero and variance σ2 > 0. Also assume that the errors are independent of the variable Degree.Linear Models代写
- Findthe least squares estimate βˆ1 for β1.
- Find the least squares estimate ξˆ for ξ = β2− β1. Is it unbiased?
- Supposeone is interested in the squared difference ζ = (β2 β1)2. Let ξˆ be as in part (b), is ξˆ2 an unbiased estimate of ζ? Prov
- Ifξˆ2 is not an unbiased estimate of ζ, provide a way to correct for the bias and give an unbiased estimate of ζ.
- Is it possible to provide an unbiased estimate of γ = β2β1 ? If yes, describe your strategy in achieving this. Note that γ can be interpreted as the absolute difference between Undergraduate and Master.
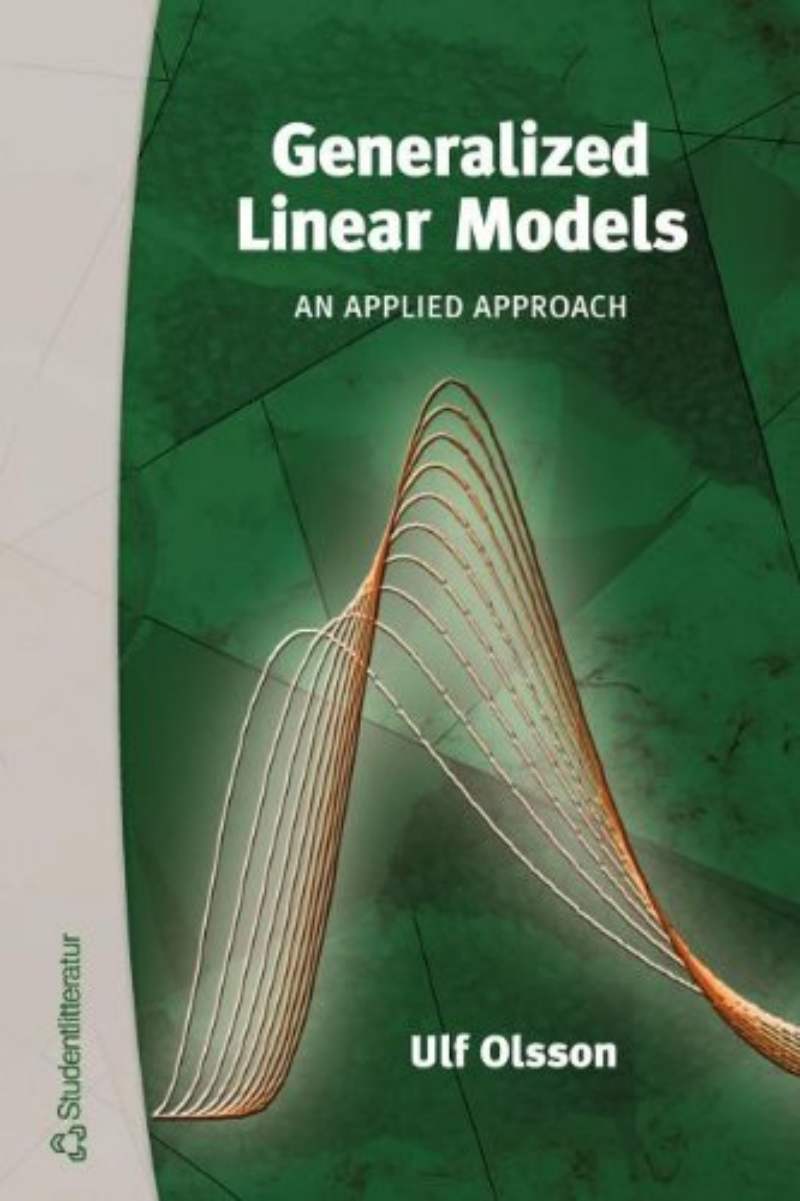
其他代写:考试助攻 计算机代写 java代写 algorithm代写 代写CS function代写 C/C++代写 paper代写 r代写 金融经济统计代写 matlab代写 web代写 物理代写