Study on Predicting Trending Events in Event-Based Social Networks
EBSN代写 Event-based social network (EBSN) is a new type of online social service. Compared with the traditional social networks
ABSTRACT EBSN代写
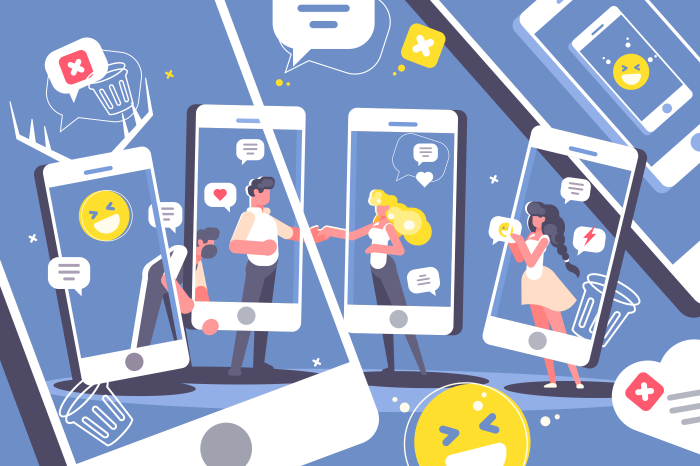
Event-based social network (EBSN) is a new type of online social service. Compared with the traditional social networks, the main feature of EBSN is that it not only includes the online social interactions (such as friends relationship, etc.), but also contains valuable offline social interactions captured in offline activities. Users can publish new event information and participate in activities they are interested in on EBSN. With rapid development and more popularity of EBSNs, predicting trending events which have many participants in EBSNs will bring great commercial benefits.
Generally, factors that influence the decisions of users to participate in an event are multifaceted. Focusing on the most important factor affecting users’ decision in attending an event, I propose an idea of a new alogorithm (i.e., TPA-ICM) to predict trending events in EBSNs. First, we calculate a user’s interest distribution according to his/her historical participating information. Then we measure the influence between users on different topic with factor graph model and TPA propagation algorithm. Finally, by utilizing classical independent cascade model of information dissemination model, we are able to predict the number of participants in new events so that trending events can be selected.EBSN代写
1.INTRODUCTION
Event-based social network is a new type of social network. With the development of science and technology, event-based social network is becoming increasingly popular. There are tons of events released on the event-based social network every day, making that the prediction of trending events in the event-based social network can bring huge commercial benefits. This chapter will briefly introduce the concept of event social network, clarify the research background, research objectives and research content of this topic, and finally give the structure of this proposal.
1.1BACKGROUND OF STUDY EBSN代写
There are many kinds of event-based social network on the Internet. The typical event-based social networks are Meetup (www.meetup.com), Plancast (www.plancast.com), and Douban City (www.douban.com/location/). Event-based social networks provide users with very convenient services such as the ability to post information on the site for a wide variety of social events (or events), whether it is informal small events (eg: simple parties, dinners, etc.), or formal large-scale events (such as large-scale concerts, technical meetings, business meetings, etc.).
And before the event (or event) formally held, the relevant information of the event can be disseminated in advance, so that users on the website Pre-event understanding of the theme, venue, ticket prices, etc., to attract more users to participate, so that events can be successfully organized. Compared with the traditional social networks (such as: Renren, Facebook, etc.), the biggest characteristic of event social networks is that it not only provides traditional online social services (such as sharing comments and photos, etc.) but more importantly, it can promote face to face Of offline social activities carried out, which indirectly strengthen the contact between users. Figure 1-1 is an example of event-based social network (from meetup).
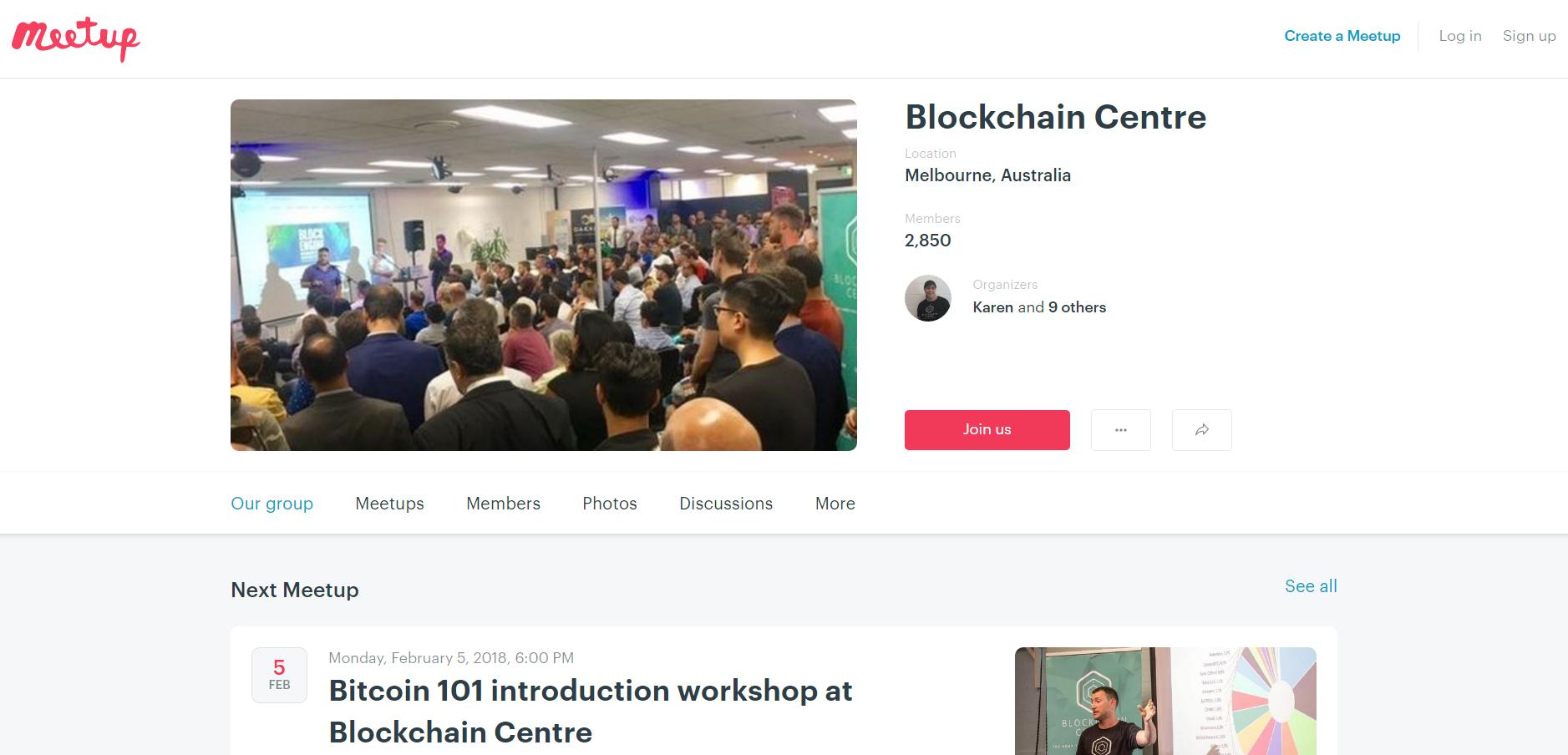
Figure 1-1 An example of event-based social network (from meetup)
1.2PROBLEM STATEMENT
Trending events are those with a huge number of participants. Forecasting trending events in the event social network can be judged by predicting the number of participants in a new event. If a new event has a high predicted number of participants (the predicted number of participants is greater than the threshold value), we consider the event to be a trending event
There are many factors that influence a user to participate in a new event for a user. Generally, there are three aspects to this effect:
1)The content of the event
For social network users, different users have different preferences, for example, some users like to listen to music, and some users are keen on watching crosstalk performances. Intuitively, the more an event’s content matches the user’s interest, the more likely it is for the user to participate in the event. EBSN代写
2)The location of the event
Obviously, users are more inclined to go to a location close to their location and convenient transportation.
3)Event organizer
A business association with a good reputation and reputation can more easily attract users to their own activities.
So that our problem is to quantify the impact of these factors to predict trending events.
1.3SIGNIFICANCE OF STUDY
Here are three perspectives to analyze the significance of forecasting trending events:
From the standpoint of the event organizer, a certain degree of accuracy in predicting the number of participants in a new event can help the organizer get ahead of time about the participation of new events, and will provide them with valuable decision support. To give a simple example: If the organizer wants to send a souvenir to each of the audience at the event, the organizer will prepare the souvenir before the event is officially held. And if the organizer knows that how many active viewers will be able to participate before the event, he/she can make a relatively accurate budget in advance, thus reducing unnecessary expenses, to maximize profits.
From the perspective of advertisers, if you know what events will become a hot spot, you can make the best choice for the delivery of ads. Because ads are served to hot events, more people can see, so as to maximize publicity, increase awareness and increase revenue.
From the user’s point of view, if the forecast of hot events can give users some effective advice. Such as reminding users whether they need to buy tickets in advance, whether they need to pay attention to the traffic conditions on the day.
2.LITERATURE REVIEW EBSN代写
2.1Independent cascade model
The independent cascade model is a random information dissemination model. It can simulate the flow of information or influence in the network (Kempe, 2013). In ICM, the process of simulation of information flow can be summarized as:
First of all, according to a certain method, we select some nodes as the initial “active node” and then iterate step by step according to the following rules:
1) When a node v becomes “active,” then it has one and only one chance to activate the node in its neighbors in a “quiescent state.”
2) It should be noted that for any node v in the “active state”, it has one and only one chance to activate its own neighbor node. The probability of successful activation depends on the influence between node v and the neighboring node.
3) If the node with “quiescent state” in the neighbor node of node v is successfully activated, then this node will be changed from “quiescent state” to “active state” and then the neighbors connected to itself will be deactivated according to the same rules node.
4) This process iterates continuously until convergence, ie: no node in ” quiescent state ” becomes a new “active node”.
2.2Factor graph EBSN代写
A factor graph is an undirected graph containing two types of nodes: variable nodes (denoted as factor graph ovals) and factor nodes (denoted as squares). The graph only contains edges between variable nodes and factor nodes (Koller, 2009). We know that a global function can be decomposed into products of multiple local functions through factorization. The relationship between these local functions and the corresponding variables is shown in the factor graph.
3.METHODS
Relevant research (Rong, 2014) has shown that the correlation between the content of an event and the interest of the user has the greatest impact on whether or not the user participates in a new event. Therefore, we designed the TPA-ICM algorithm, which is the algorithm that predicts the hot events in the event social network, which is the most influential factor for the users to participate in the new events.
In this trending events prediction problem, factor graph and independent cascade model could be combined to solve this problem. First, factor graph model could be used to simulate the social network, then according to a Topical Affinity Propagation (TAP) algorithm proposed by Jie Tang in 2009, the influence distribution could be quantified. After that, the influence distribution could be used in independent cascade model to how many user nodes could be activated in the social networks. The whole model is called TAP-ICM model. EBSN代写
3.1 Influence quantification
Figure 3-1 Topical Factor Graph
In Figure3-1: T represents the number of topic; z represents topic; v represents the node; represents the node which has the hugest influence on node i in topic z; is the hidden vector of node v, which is composed by all (1<=Z<=T).
In this Topical Factor Graph model, we have three feature functions: 1)Global feature function h(vi , yi , z) ; 2) Edge feature function f (vi , yi , z); 3)Node feature function g(vi , … , yi , z).
According to the theory of factor graph, we hope that the model we constructed will be able to fit the data we observed. The usual method is to maximize the likelihood function of observed data (ie, Maximum likelihood estimation). Based on the established model and the feature functions, we define the observed likelihood function as follows:

When training the TFG model, we can find Y=, y1, …, to get the largest P (v, y) by observing the likelihood function P.EBSN代写
After that, we can use the Basic TAP Learning Algorithm to find the influence between adjacent nodes in a social network G under different topics z, the detailed method is described in Jie Tang’s paper.
3.2 Influence propagation and prediction EBSN代写
Once we get the quantification of influence, we can use independent cascade model to simulate the influence propagation in event-based social networks:
- set the threshold number of participants in the hot event φℎot;
- Given a new event new_e, calculate its probability distribution over T topics using LDA based on its content information;
- Find the number z of the highest probability distribution in T topics;
- According to the theme z, select the influence network corresponding to Gz;
- Choose the participant users who want to join within two days after the new_e message is published as the initial “active node” collection for the IC model;
- Use IC model’s information propagation algorithm to obtain the user nodes that may participate in new_e, that is, the user nodes in the last “active node” collection. The number of users in the last “active node” collection is the predicted number of participants eva_num, If eva_num ≥ φℎ, it is judged that this event is a hot spot (trending event). ,
4.CONCLUSION EBSN代写
In this article, our goal is to predict the number of participants in a new event in the event-based social network (EBSN). Taking the content of the event as the main factor driving the user to participate in the event, the TPA-ICM prediction algorithm is designed using the influence of the user’s interest. TPA-ICM prediction algorithm includes two main models: Topical factor graph model and independent cascade model.
The Topical factor graph model calculates the influence among users under different topics based on the user’s friend relationship.
The independent cascade model can simulate the spread of information flow in social networks, and thus predict the likely number of participants for a new event.
Trending event prediction and influence propagation are two important research areas in social network, I think these 2 area’s study could fit in the ongoing research about social network in your lab.
5.Future Work
There is still some future work need to be done in the research of this topic. There is only one factor to consider when determining the user participate the event or not in this proposal. In reality, there are many factors that can influence him to participate in a new event. Beside the topic information of the event, the organizer of the event is another important factor.EBSN代写
In the future work, In order to utilize the organizer information, we can collect the event history information of organizer, then process it with a RNN or LSTM deep neural network. After that, we can get a general feature of the whole history information of the organizer, this feature could also be used in judging the event is a trending event or not.
6.PLAN
In my phd phase, I will focus on the research about the social network’s influence propagation and relationship analysis, I would treat it as my overall goal in my phd. Predicting trending events is a problem comprised influence quantification and information propogation.
It is a meaningful and significant research topic in social network, I would like to try solving this problem in my early phd phase. The first problem of this topic is to model the whole social network and quantify the influence between people in the social network, I would like to use the probabilistic graphical model to solve this problem (ie: factor graph).EBSN代写
Using probabilistic graphical model, we could get the global probability distribution of the network, after that, we could calculate the margin probability of each node. After modelling this network, we could use it to quantify the influence, then continue to analyze the information propagation between nodes.
In this proposal, I proposed a new blend model for predicting trending events. It includes a factor graph model and Independent cascade model, I would focus on adjusting and optimizing this model to solve the trending events predicting problem in my later research in your laboratory. Moreover, I could take part in other research in your laboratory about social network, such as Social, cultural, and behavioral modeling, etc.
References
Kempe D, Kleinberg J, Tardos É. Maximizing the spread of influence through a social network[C]//Proceedings of the ninth ACM SIGKDD international conference on Knowledge discovery and data mining. ACM, 2003: 137-146.
Rong Du, Zhiwen Yu, Tao Mei. Predicting Activity Attendance in Event-Based Social Networks: Content, Context and Social Influence. UbiComp’14, September 13 – 17 2014:425-434.
Koller D, Friedman N. Probabilistic graphical models: principles and techniques[M]. MIT press, 2009.
Jie Tang, Jimeng Sun, Chi Wang, Zi Yang. Social Influence Analysis in Large-scale Networks. KDD’09, June 28–July 1, 2009, Paris, France.
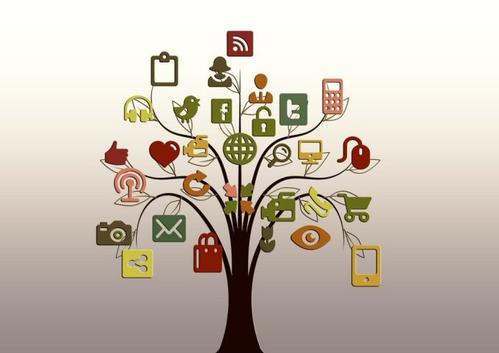
更多其他: 数学代写 assignment代写 数据分析代写 程序代写 算法代写 经济代写 代写CS C++代写 java代写 r代写